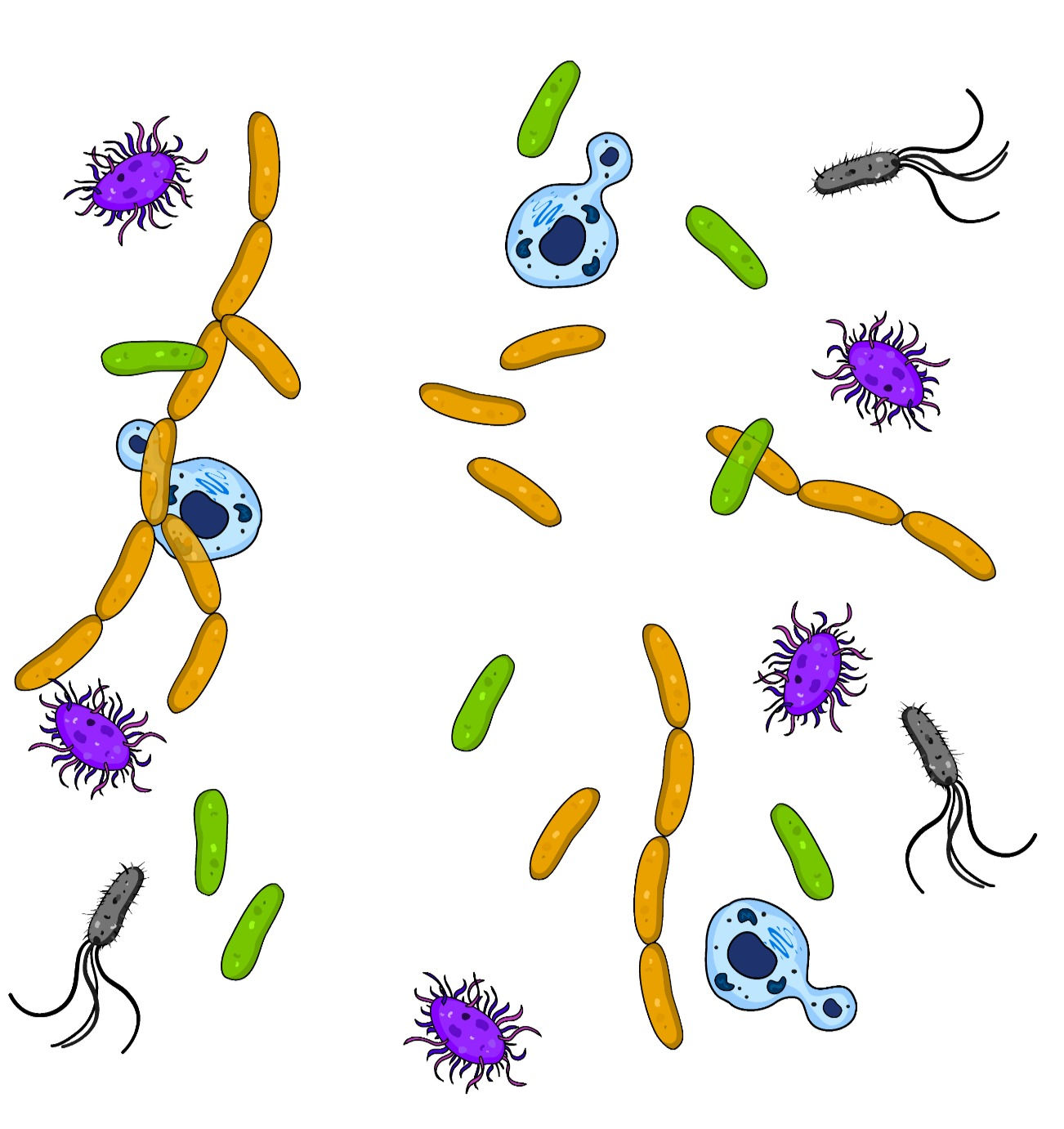
Research Summary
Evolution of cooperation and cheating
Cooperators pay a cost to produce public benefits, while cheaters exploit these benefits. How has cooperation evolved and been stabilised? Using an engineered yeast cooperative community, we are examining how cooperation might evolve, step-by-step, from inception. We are also investigating how community robustness (the ability of a community to survive various perturbations) might change during evolution.
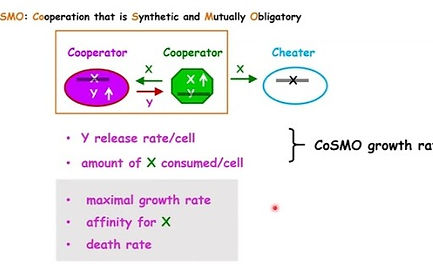
Quantifying microbial phenotypes: from cells to communities.
Community properties, such as community robustness and community functions (biochemical activities of a community), arise from interactions between community members. A quantitative understanding of how interactions lead to community properties will allow us to manipulate community properties. Taking advantage of simplified communities with known metabolic interactions, we have been developing methodologies to quantify interactions. Quantified interactions are used to parameterise mathematical models to predict community properties, which are then experimentally tested. When predictions deviate from experiments, we improve our quantification methodology or discover new biology. We have applied this approach to quantitatively understand sulfide gas-mediated microbial interactions. We also attempt to predict microbial phenotypes when interactions are not known by building quantitative models using model selection and parameter inference.
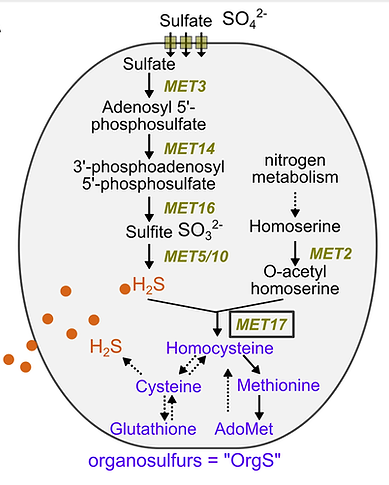
Artificial selection of microbial communities
Multispecies microbial communities often display useful community functions such as pharmaceutical production and waste digestion. Community functions arise from species interactions, but interactions are difficult to identify. To improve a community function, one can perform artificial selection on whole communities: Many communities are repeatedly grown such that mutations may freely arise. Communities with the highest desired function are “reproduced” where each is randomly partitioned into multiple offspring communities for the next cycle. However, previous community selection efforts have often encountered difficulties. Using computer simulations, we have identified some failure modes, and predicted effective strategies. We will experimentally test these strategies using a liquid-handling robot to perform artificial selection on a variety of communities.
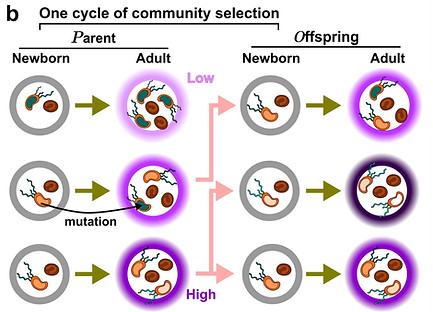
Causal inference in ecology and evolution
Although mechanistic knowledge in biology is rooted in manipulative experiments, the perturbation of living systems can encounter practical or ethical barriers. A parallel strategy is to infer causal knowledge by analysing time series data. For example, by observing microbiome data from a patient, we would like to formulate hypotheses such as “perturbing the abundance of species X can perturb the abundance of species Y”. To increase the accuracy of causal hypotheses, we are developing statistical methods for diagnosing and overcoming limitations associated with current approaches. We also work on predicting the effect of mutations in different genetic and environmental backgrounds.
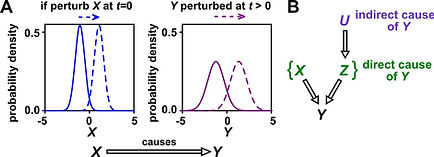